Customer support tickets themselves are rich, lengthy, and come in high volumes.
Which makes them both a goldmine of customer insight and a ruthlessly difficult task to tag and categorize.
Natural language processing is one effective solution for efficiently understanding and categorizing support tickets without significant manual analytics.
In this article, we’ll answer three core questions:
1/ What types of natural language processing are there and how do they categorize differently?
2/ Does Zendesk have a good quality natural language processing engine built in?
3/ Why type of NLP is essential to accurate ticket tagging?
At SentiSum, we build a critical ticket categorization for Zendesk (Find us on the Zendesk Marketplace).
We use cutting edge machine learning-based categorization technology which applies accurate, detail tags to support tickets in real-time.
In this article, you'll also learn more about how our integration improves upon what Zendesk has already and the ways you can use case our ticket tags to automate workflows in Zendesk and more.
What is natural language processing?
NLP is how we can get computers to understand language—speech and text.
One of the main benefits of having a computer understand human language is that, unlike humans, they are incredibly fast, consistent and unbiased in their approach.
Think of a human reading a document and noting down elements of meaning within that document. It’s like that, but being a computer, it’s not limited by reading and writing speed and can read and categorise millions of documents in seconds.
So when they process text in large batches—let's say 100,000 support tickets—it can be done in an instant, and the output is relatively very actionable.
There are three distinct types of NLP (here in chronological order of development):
1/ Keyword extraction
Providers like Zendesk and Intercom provide only a simple keyword extraction tool built in to their solution.
A keyword extraction system follows very specific rules. For example, If the word ‘refund’ is present in a piece of text, the system will tag it as the topic is ‘refund’.
Applied to thousands of support queries, this method has obvious flaws.
What if a customer says ‘can I get my money back?’ instead of ‘can I get a refund?’. It wouldn’t tag the topic correctly.
What if a customer said, ‘I don’t want a refund, but I’m not happy.’ This would be incorrectly tagged ‘refund’.
The result is that ‘keyword extraction’ can only help to provide an inaccurate, high-level categorisation.
Getting meaningful results are difficult. For example, uncovering an actionable, insightful statement like "2,340 support queries this month were on the topic of Paypal payment failure." would take a significant amount of manual analysis on top of keyword extraction.
2/ Rule-based NLP
Rule-based NLP has improved accuracy relative to keyword extraction.
Unlike keyword extraction, it doesn't only look for the word you tell it to, but it also leverages large libraries of human language rules to tag with more accuracy.
For example, the library of rules tells the computer that ‘liberty’ and ‘freedom’ mean the same thing...so tag both.
A rule-based NLP system simply follows these rules to categorise the language it’s analysing.
As you can imagine, if the rule doesn’t exist, the system will be unable to ‘understand’ the human language and thus will fail to categorise it.
Unfortunately, this means accuracy is dependent on the rules provided. When you have a unique business environment, and want detailed results, it is practically impossible to give the software all the rules required.
3/ Machine learning-based NLP
SentiSum is a machine learning-based NLP system.
A machine learning-based NLP system relies on more modern ‘statistical inference’ techniques.
It's more intelligent and understands speech and text in a similar way to how humans do.
Once it’s learned to understand human language in a particular environment—say, the legal world—it can infer the meaning of misspellings, omitted words, and new words without a human setting up a new rule.
Machine-learning also learns the patterns between phrases and sentences and is constantly optimising and evolving itself so that it’s level of accuracy is getting ever closer to reality.
After some upfront training by SentiSum, we could let it loose on a data set and it would categorise it with increasingly higher accuracy.
Let’s take a look at all three in action:
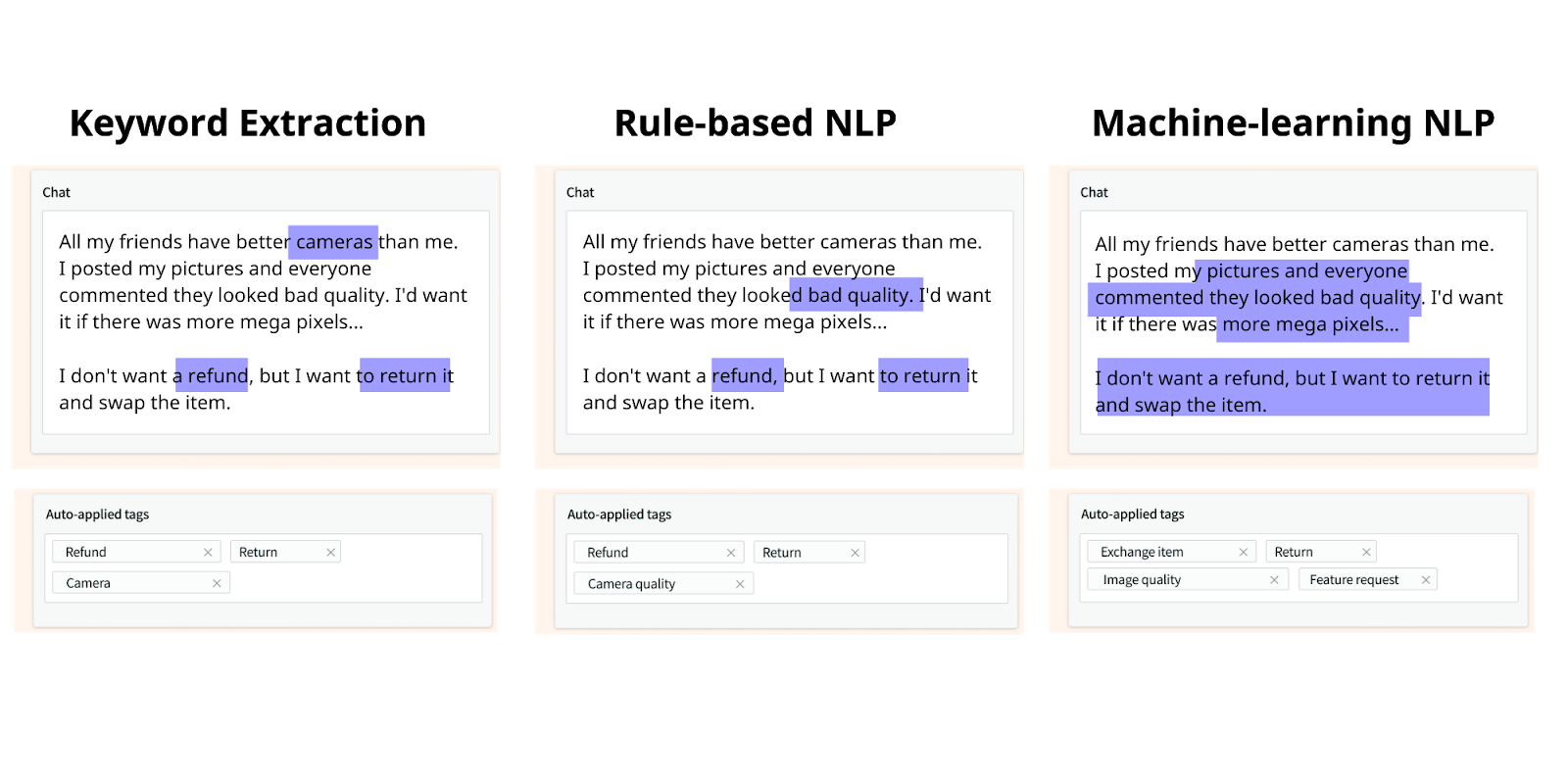
As you can see, keyword extraction and rule-based NLP is simplistic and inaccurate. Over thousands of support queries the impact is enormous. Machine learning is more intelligent with its tagging, providing much greater accuracy.
Keyword extraction: Blindly tags any keyword it’s told to.
Rule-based: Blindly follows more advanced rules. It might know ‘adjective’ + ‘noun’ indicates a customer's opinion about that noun. So ‘bad’ + ‘quality’, indicates a quality issue for another noun mentioned, ‘camera’, let's tag the topic with ‘camera quality’.
Machine-learning: Doesn’t blindly tag keywords or ‘rules’. It infers meaning based on patterns between words and the wider context of the sentence and paragraph they sit within. In the above example, the last sentence says ‘I don’t want a refund’, machine-learning is the only one to understand this nuance and not tag the ticket with the topic ‘refund’.
Find a lengthy and coherent description of rule-based vs. machine learning-based systems here.
Why is Zendesk machine learning integration essential to uncovering support ticket insights?
Customer support ticket logs are:
- High volume—Probably the highest volume and frequency of customer feedback available in most companies. Many of our customers have 50-100k new conversations each month.
- Rich—The conversations are usually lengthy and detailed explanations of the customer's issue.
Which means they are high value and worth analysing properly.
However, they are also:
- Raw
- Unstructured
- Unpredictable in length
Every customer expresses their issue in a different way. And some issues are much more complex than others.
Combined, that means manually analysing tickets is near impossible, especially in a useful level of detail and granularity.
The complexity of customer support queries also means rule-based NLP and keyword extraction is not fit for the job—there are just too many spelling and grammar mistakes and variations of ways to express an issue; too many ways they will tag incorrectly.
Zendesk makes use of a naive rule-based system to tag and categorize tickets. This means those tags are high level such as "website_issue", "presale_enquiry" and so on. They do not mention the specific reason for customer contact.
Using Zendesk’s in-built tool means that:
- Tags will frequently be incorrect
- Tags will be generic and high level
- Truly understanding reasons for contact will still require significant manual data handling to get accurate insights
Whereas machine learning-based automated ticket tagging will ensure:
- Tags are high accuracy
- Tags are specific to the reason for contact. Not ‘checkout issue’, but ‘discount code failed’.
- Analysis and reporting requires little to no manual data handling.
SentiSum’s Zendesk integration is machine learning-based. We also bring a new, useful level of ticket tagging to the equation called: hierarchy.
The easiest way to explain tagging hierarchy is with a comparative example.
Zendesk text analytics, the in-built solution, may tag a ticket with 'missing item'. Whereas, SentiSum would have 'missing item' as a top level topic and your products as subtopics. SentiSum would tag the ticket with 'missing item' AND 'product name', so you can start high level and deep dive to the root cause of the issue.
With machine learning, SentiSum brings greater detail and accuracy to the ticket tagging process, with hierarchy, SentiSum brings greater usefulness.
SentiSum's Zendesk NLP integration
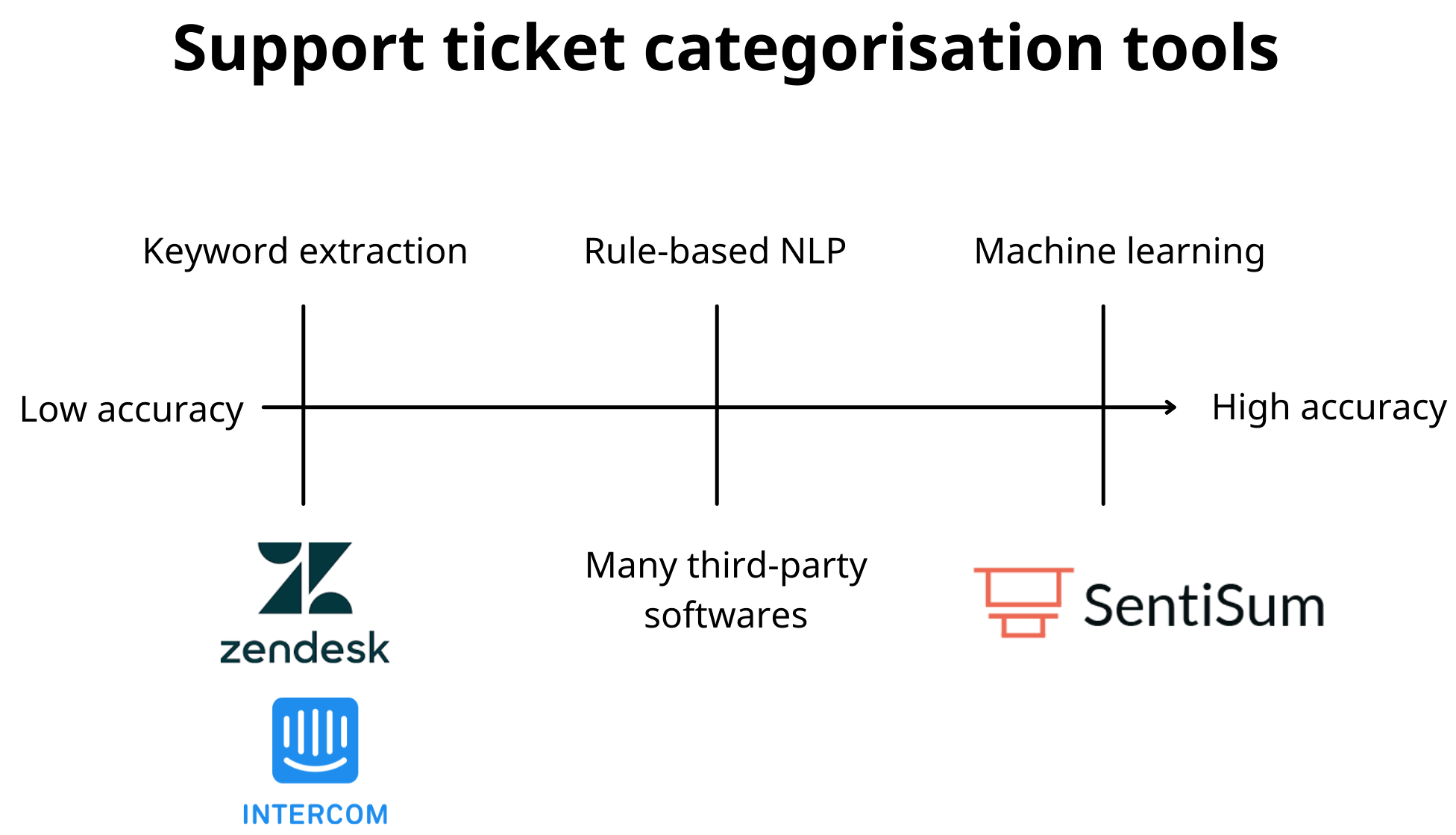
We built a robust Zendesk automated tagging engine around our proprietary machine learning-based natural language processing.
As your support queries enter the queue, they are immediately digested and categorised at a granular level.
We've built an easy-to-use insights dashboard which makes deep diving your ticket tags and reporting on them each week a simple task.
Tags are also pushed back to Zendesk, so you'll see them appear along the side the ticket. Using our advanced tags, you'll be able to route tickets based on their topic and prioritise tickets when a customer is particularly angry or a topic is particularly important.
Watch a video of our dashboard here. Learn more about our Zendesk automations here. And learn more about ticket routing here and ticket prioritisation here.
We pride ourselves on:
- Accuracy of insight: Tags are highly accurate.
- Granularity of insight: Finding insight you didn't know about.
- Consistency of application: We build you a custom algorithm just for your data.
Which is why we offer you a 30 day free trial to prove it works on your data. (book a meeting with us here to sign up).