Ever wish you could read your customers' minds? Your customer conversations come close.
They hold the key to understanding why customers reach out in the first place, and how they feel about each issue and interaction.
You can then use this information to truly improve customer experience, reduce churn, and increase revenue.
Historically however, this data has been difficult to extract and analyze because of its sheer volume and qualitative nature.
But with artificial intelligence (AI), you can now analyze every customer conversation accurately, and at scale.
In this article, we explain how AI-powered sentiment analysis software works and explore five ways you can use it to analyze your conversation data.
Jump to:
- What is customer sentiment analysis AI software, and do you even need it?
- 6 types of sentiment analysis (and their use cases for each)
- 5 ways you can analyze your customer data using AI
What is Customer Sentiment Analysis AI Software? And Do You Even Need It?
Customer sentiment analysis software helps you understand how customers feel about your products, services, or brand by analyzing customer interactions across various channels using AI.
Put simply, it’s like having a smart assistant that reads through all your customer emails, chats, reviews, and social media comments to tell you how your customers feel and why they feel that way.
Here’s how it works on an AI-powered customer sentiment analysis tool like SentiSum:
- Data Collection: Gathers unstructured data from multiple sources like emails, chats, social media, and phone calls.
- Natural Language Processing (NLP): Uses NLP to understand the context and meaning of the words and phrases used by customers.
- Machine Learning: Applies machine learning algorithms to identify patterns, trends, and sentiments in the data.
- Sentiment Analysis: Categorizes sentiments as positive, negative, or neutral and highlights key emotions and issues.
- Visualization: SentiSum’s dashboard shows actionable insights to improve customer experience, product development, and overall business strategy.
This video walks you through the steps:
On SentiSum, you can dive deeper into individual messages and agent performance to further understand what’s driving trends in customer sentiment.
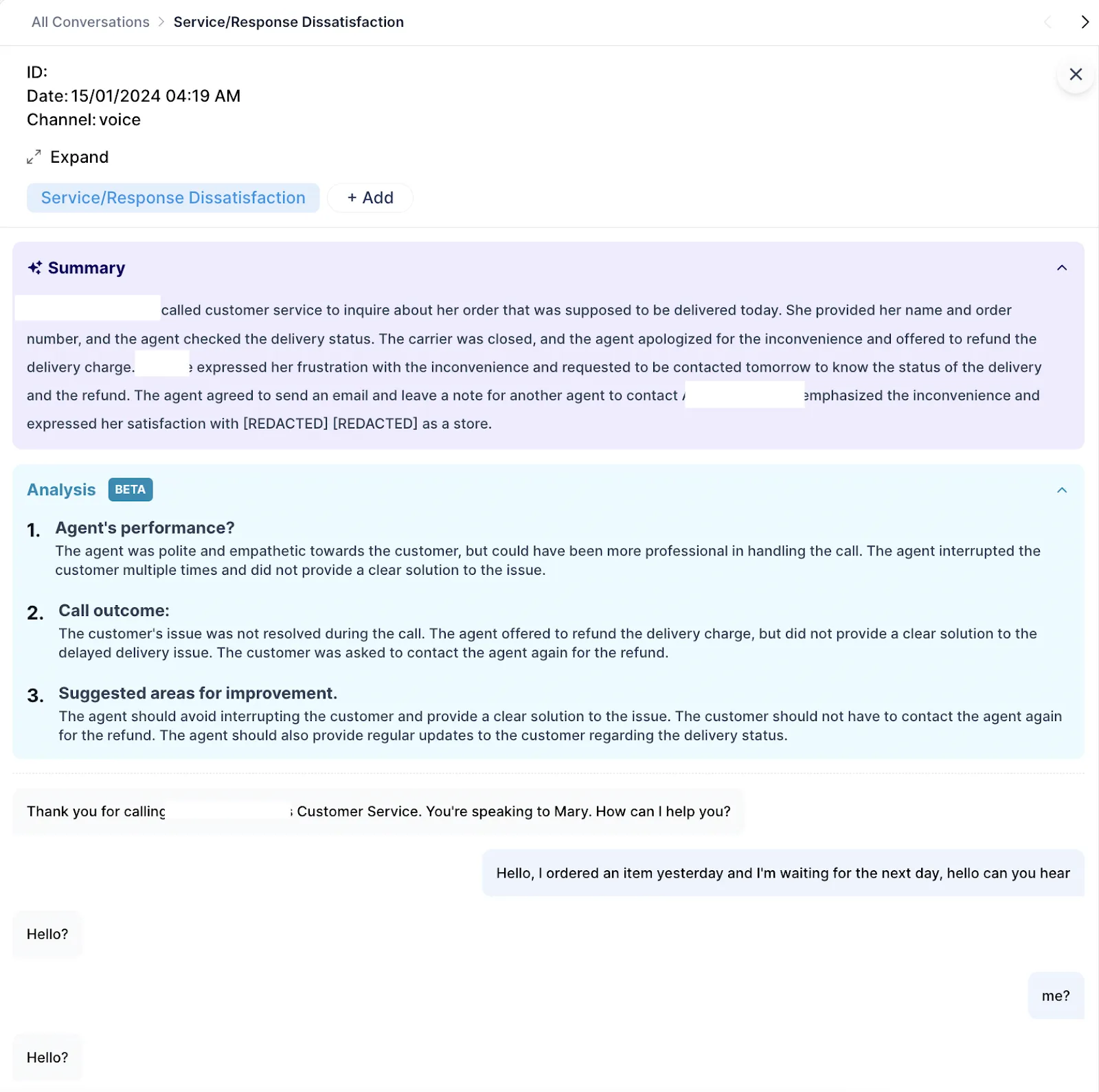
Want to understand the root causes of issues immediately? No problem.
You don’t need to read through transcripts or waste time interpreting data.
SentiSum’s GPT-powered AI assistant called Dig In, gives you summarized answers to any question about your customer conversation data.
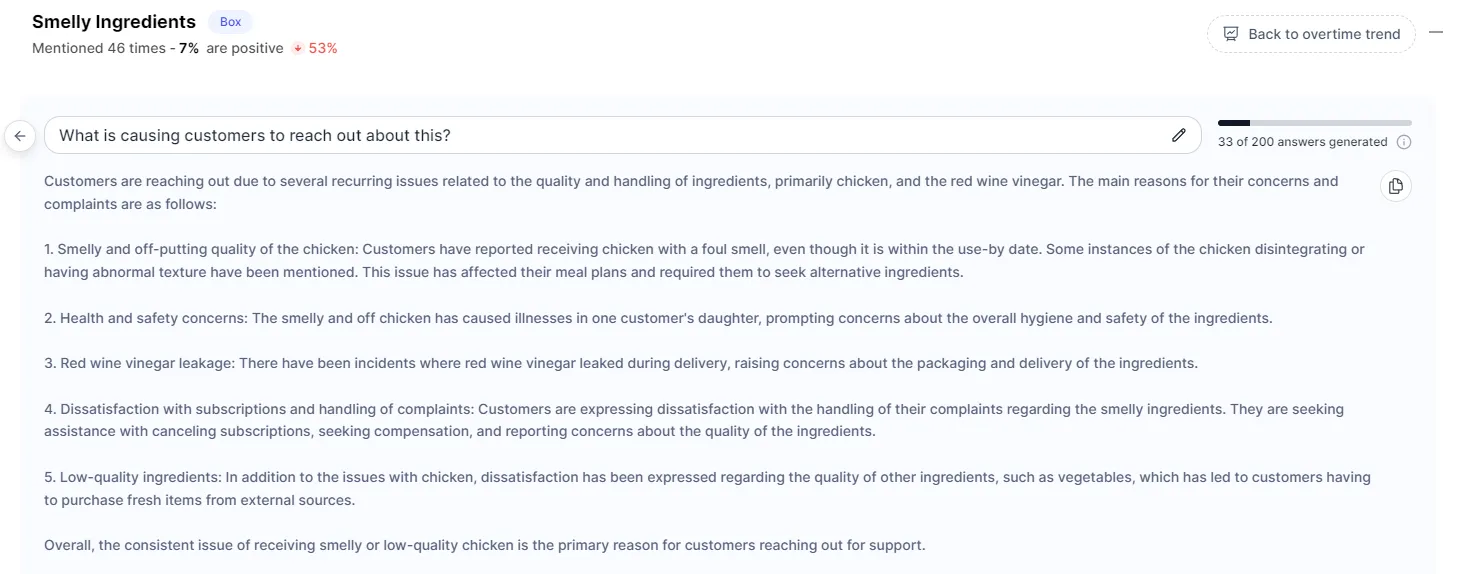
Now, let’s answer this question → Do you need customer sentiment analysis software?
Yes, if you’d like to see considerable improvements across key business metrics.
Data from Forrester revealed that organizations with “mature deployments” of AI-powered analytics software saw the following:
- Increase in NPS scores (63%)
- Higher conversion rates (50%)
- Higher revenue (56%)
- Increase in order size (40%)
- Increase in overall job satisfaction among support agents (50%)
Grab a demo wit SentiSum below to see how the software works in action.

So, what kind of customer sentiment analysis types are there, and how can you apply them to your customer conversations? Let’s find out.
6 Types of AI Customer Sentiment Analysis
Here are the primary types of customer sentiment analysis AI:
- Lexicon-Based Sentiment Analysis
- Machine Learning-Based Sentiment Analysis
- Hybrid Sentiment Analysis
- Aspect-Based Sentiment Analysis
- Visual Sentiment Analysis
- Multimodal Sentiment Analysis
Let’s look at each in more detail.
Lexicon-Based Sentiment Analysis:
This method uses a predefined list of words (lexicon) to determine sentiment based on the presence of positive or negative words.
But it doesn’t consider the context in which words are used, leading to potential misinterpretations of sentiment.
Machine Learning-Based Sentiment Analysis:
Machine learning uses algorithms to learn from data and classify text based on sentiment.
This method is highly effective in handling large volumes of data and improving accuracy over time as more data is processed.
It can also capture nuances and complexities in language (which other methods can’t).
One shortcoming of this is that it requires a large amount of labeled training data and significant computational resources to build and maintain accurate models.
Hybrid Sentiment Analysis:
This hybrid method combines the strengths of lexicon-based and machine learning-based approaches, providing a balance between context understanding and computational efficiency.
However, it may be complex to implement and can inherit limitations from both individual approaches.
Visual Sentiment Analysis:
As the name suggests, this method analyzes visual content (images and videos) to determine sentiment based on facial expressions, colors, and other visual cues (think word cloud).
It’s useful for social media and brand monitoring but is limited by the current capabilities of image recognition technology and may struggle with ambiguous or complex visuals.
Multimodal Sentiment Analysis:
This method combines multiple data types (text, audio, and visual) to assess sentiment, providing a more accurate sentiment analysis by considering different modes of communication.
However, it’s highly complex to implement and requires integration of various data sources and advanced analytical capabilities.
Aspect-Based Sentiment Analysis:
This is a more granular approach to understanding customer sentiment.
Instead of assessing an entire piece of text as positive, negative, or neutral, it breaks down the text into smaller units called aspects (aka topics and subtopics) related to specific features of a product or service.
For each aspect, it evaluates the sentiment expressed.
This method allows you to pinpoint exactly what customers like or dislike, providing more detailed insights into customer preferences and areas for improvement.
For example, in the image below, our sentiment analysis AI is integrated directly into the Zendesk of a video game company.
All their customer conversations are now categorized with topics and subtopics ("aspects"), and each of those topics is analyzed to understand its sentiment.
The result?
The gaming company now understands issues causing their customers to reach out (and the impact of them).

They now know that Game Froze has driven 46,000 customers to contact customer service and only 2% of those were labeled positive.
This data can be used to:
- Encourage other teams to act with more urgency.
- Identify projects to prioritize.
- Auto-route tickets causing particular negative sentiments to an “urgent” team.
So, where can you apply AI customer sentiment analysis, and what kind of insights can you get from it?
5 Ways AI Customer Sentiment Analysis Transforms Your Feedback Channels into Actionable Insights
You can analyze data from all these channels using AI sentiment analysis tools:
- Phone calls
- Surveys
- NPS
- Customer reviews
- Support tickets
1. AI Sentiment Analysis on Phone Calls
Traditionally, analyzing phone calls has proved challenging due to the qualitative nature and sheer volume.
But with AI-powered sentiment analysis software like SentiSum, you can easily track and analyze your voice data.
In a nutshell, here’s how it works:
- First, the software records each support call.
- It then uses speech recognition technology to transcribe the spoken words into text.
- A NLP model then analyzes the transcribed text, uncovering key topics and sentiment.
Then, SentiSum’s speech analytics dashboard shows you key insights like:
- Total call volume
- Highest-volume call topics
- Topics that have increased and decreased in volume
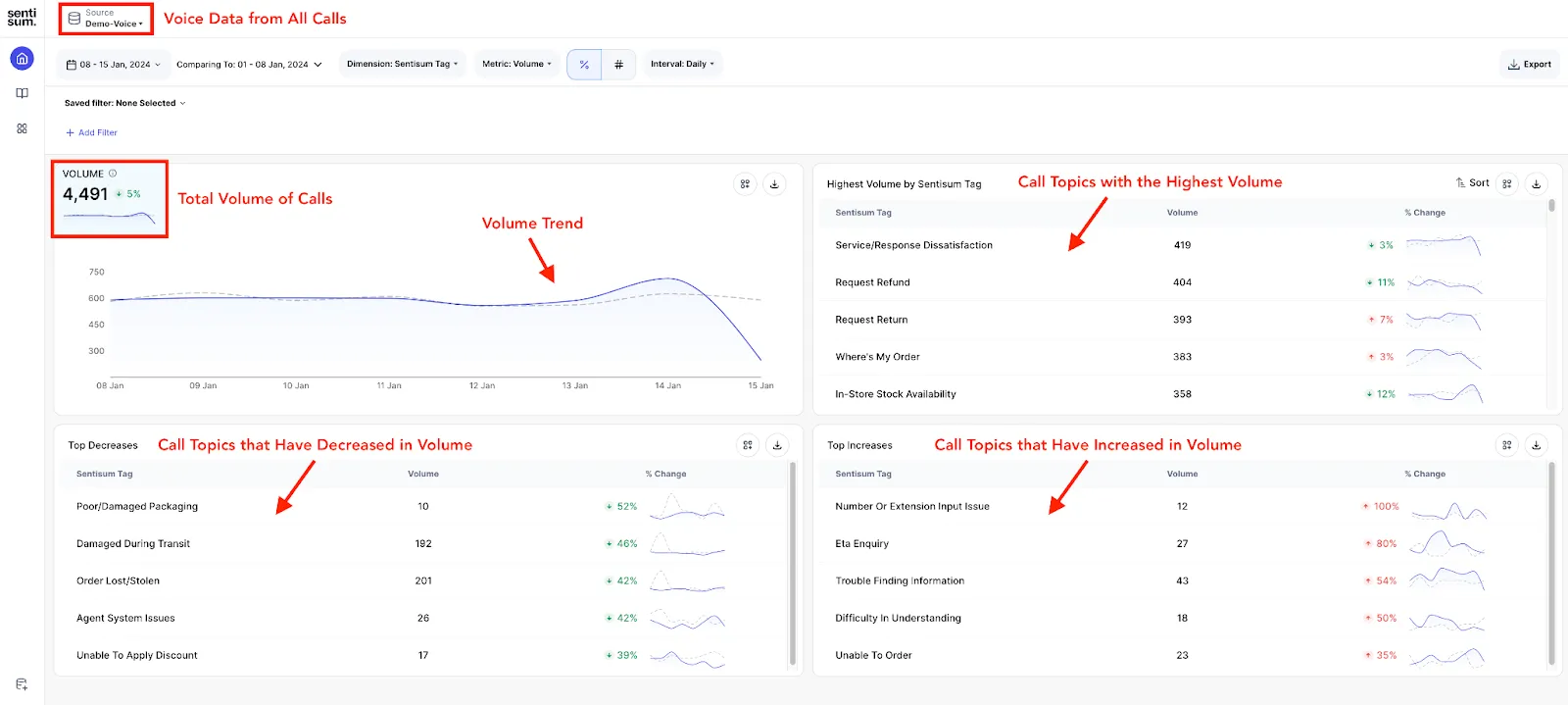
When you dig into details for individual calls, you’ll see:
- Topic (reason for contact): The main reason(s) why customers are calling, such as billing issues, technical support, or product inquiries.
- Sentiment: The overall sentiment of the call to determine whether the customer’s tone is positive, negative, or neutral.
- Urgency: How pressing the issue is. This helps your team prioritize which issues need immediate attention.
Here is an example of a customer support call about a delayed delivery that was analyzed in SentiSum:

In addition to the full transcript of the call (which is available on the right-hand side of the dashboard), you can see an AI-generated summary of the call and an analysis of the support agent’s performance.
In this particular instance, we know that the customer’s issue wasn’t resolved on the call.
However, she was offered a refund on the delivery charge and the promise of a follow-up contact about the delivery and refund status.
SentiSum automatically marked the conversation with the following topic tags:
- Order Not Delivered
- Escalation Request
- Request Refund
- Where’s My Order
- Agent Suggested to Call Back
This means for each issue, you have a granular record of what’s going on, what customers think and feel, and how you can improve their experience.
The customer’s negative sentiments (frustration, inconvenience) and positive sentiments (satisfaction with the store overall) have also been noted in the summary, as well as “Suggested Areas for Improvement” for the agent.

This means you can track the performance of each individual agent and train them to serve customers better.
Tip: Check out this 30-second video for a quick overview of how voice call sentiment analysis works in SentiSum.
How AI sentiment analysis on speech improves CX:
✅ Reduced average handle time: AI can quickly reduce the time to resolve customer pain points by identifying common issues and providing customer service agents with relevant information.
✅ Cost savings: Organizations that leverage speech analytics saved 20% to 30% in costs, according to data from McKinsey & Company.
✅ Improved CSAT scores: McKinsey & Company data revealed organizations saw CSAT scores go up by 10% or more after leveraging AI speech analytics to address issues more effectively and empathetically.
✅ Proactive issue resolution: Organizations can address problems before they escalate by identifying trends and common issues over time.
✅ Stronger sales: Increased customer loyalty and higher sales can flourish with enhanced customer satisfaction and quicker issue resolution.
2. AI Sentiment Analysis on Surveys
Traditional feedback surveys often miss the nuances expressed by customers, making it difficult to comprehensively understand customer experience.
AI-powered software like SentiSum has the ability to analyze open-text survey responses and turn them into actionable insights for its users.
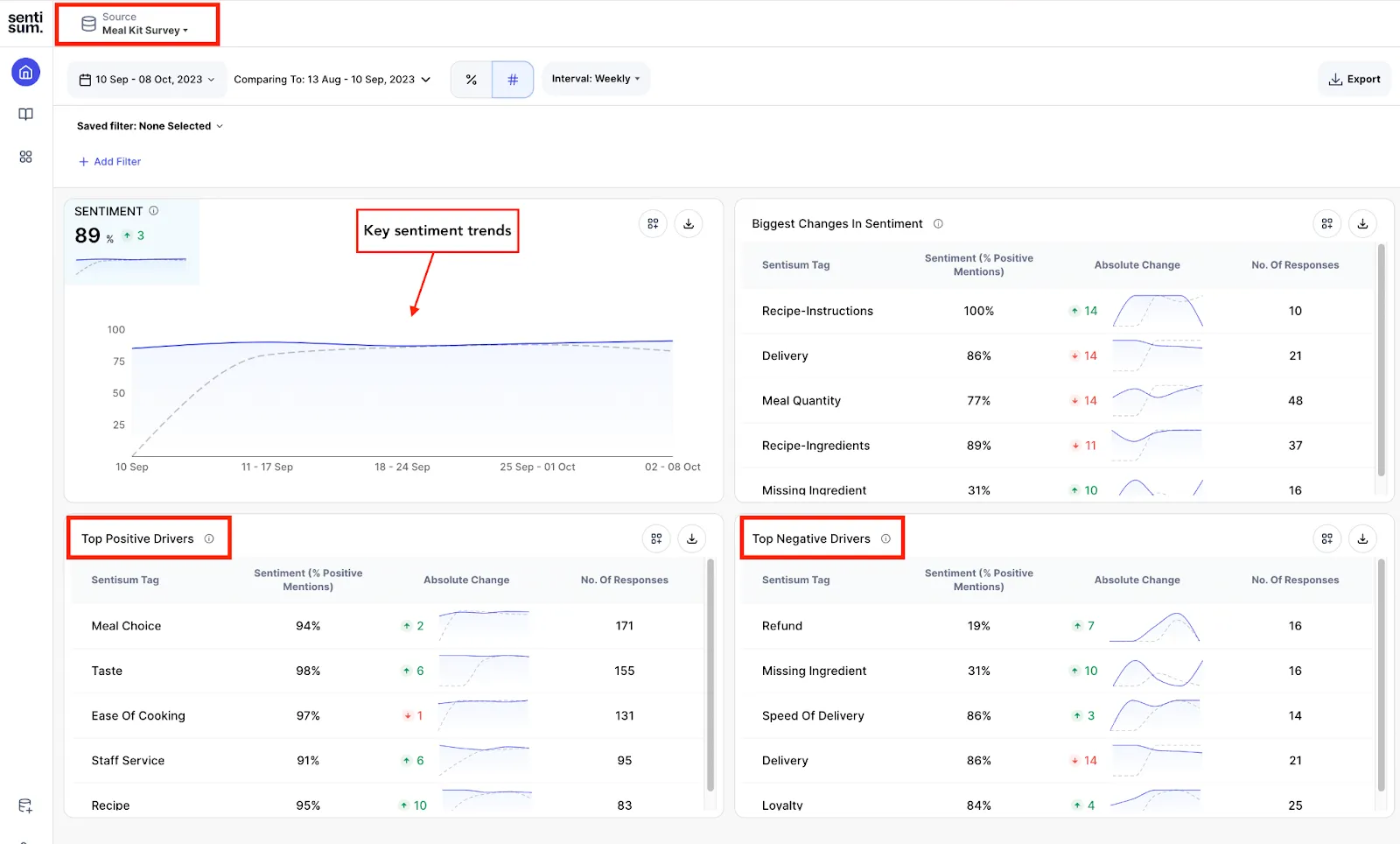
On SentiSum’s survey text analysis dashboard, you can see key trends and insights such as:
- Trends in sentiment over time, including the biggest changes.
- Top positive and negative drivers of survey scores/responses.
Individual survey responses are classified by topic, sentiment, intent, and urgency, which can be especially useful if the customer provided a free-text response explaining their score.
(Don’t worry if they didn’t, though: With SentiSum, you can cross-reference survey data with other customer conversations to piece together a better picture of what may have gone wrong for low scores.)
How AI sentiment analysis on surveys improves CX
✅ Improved CSAT: Organizations can address issues quickly and more effectively to improve overall satisfaction.
For example, Lakrids by Bülow improved its 5/5 CSAT rating by 9% from the first half of a year to the latter half, just by improving its customer support operations based on SentiSum insights.
✅ Proactive improvements: By identifying common themes and trends, organizations can make proactive changes to improve products and services before issues escalate.
✅ Reduced churn: Understanding and addressing customer concerns promptly reduces churn rates and increases customer loyalty.
Research by Esteban Kolsky, CEO of thinkJar, found that nearly 70% of customer churn is “preventable if the customer issue was resolved at the first engagement.”
3. AI Sentiment Analysis on NPS
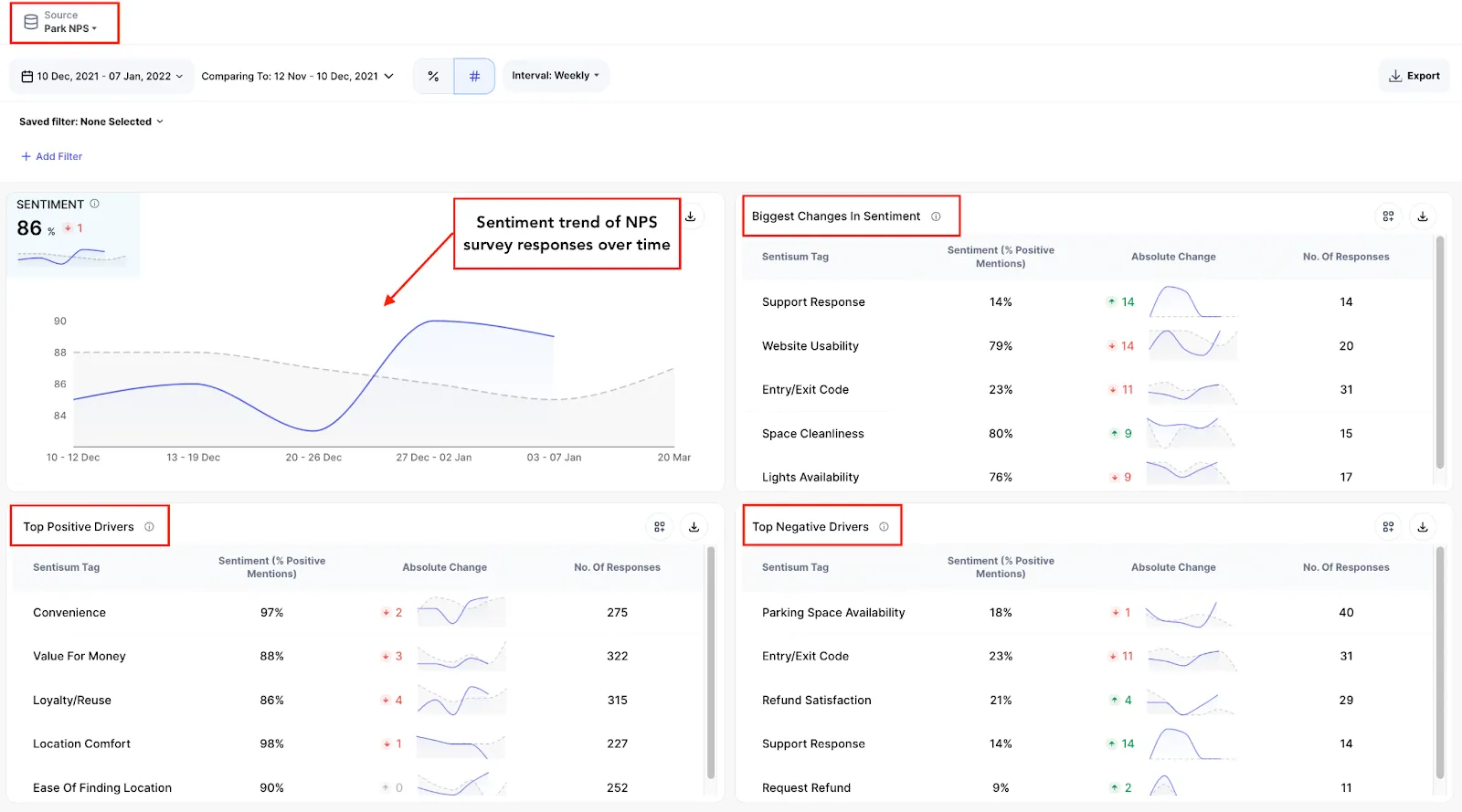
You can’t improve NPS just by looking at the score.
You need to analyze customer conversions and dig deep into the key NPS drivers so you can start to understand, and improve it. (We wrote about this in detail here.)
On SentiSum, NPS trends like response sentiment over time, biggest changes, and top positive and negative score drivers are all displayed in a convenient, easy-to-read dashboard.
You can also cross-reference a customer’s NPS response with other communication channels to better understand what was happening with that customer when they gave that score.

In the above example, AI-powered sentiment analysis gives you a more granular look at the same customer’s NPS survey response compared to a customer service ticket from around the same time.
This not only labels the survey response as “detractor,” but gives further insight into the customer’s specific issues (audio quality) and sentiment (negative).
How AI sentiment analysis on NPS improves CX
✅ Higher NPS scores: Companies can convert detractors into promoters by understanding and addressing the reasons behind detractor scores.
✅ Increased revenue: Higher customer satisfaction typically means greater retention rates.
According to HubSpot, a mere 5% increase in customer retention can boost revenue by up to 95%.
✅ Organic, targeted CX initiatives: “Text analytics [of NPS] has allowed us to focus on key areas where we can improve customer experience organically, versus trying to improve a bunch of things and hoping that improves the customer experience,” Kellan Williams, Customer & Quality Analytics Manager at Safelite, said in an interview with Customer Experience Magazine.
📖 Read more: NPS Sentiment Analysis: How To & 6 Ways It Drives Change
4. AI Sentiment Analysis on Customer Reviews
A manual analysis of customer reviews is not only time-consuming but can also cause you to overlook important insights that help you understand customer sentiment.
By using AI-powered software like SentiSum to analyze your reviews, you get a much more complete picture of what customers are saying when they leave feedback about your company.

SentiSum’s easy-to-read dashboard gives you useful insights into sentiment trends from your customer reviews (including changes over time), as well as the top drivers of positive and negative reviews.
It pulls these insights from automatic analysis of every review across every channel you integrate.
When you drill down into an individual review, you’ll see tags for topics or key themes (e.g. product quality, customer service, specific features) and the sentiment (positive, neutral, negative).
You can even sort your data by topic to see more detailed insights about reviews mentioning that term.
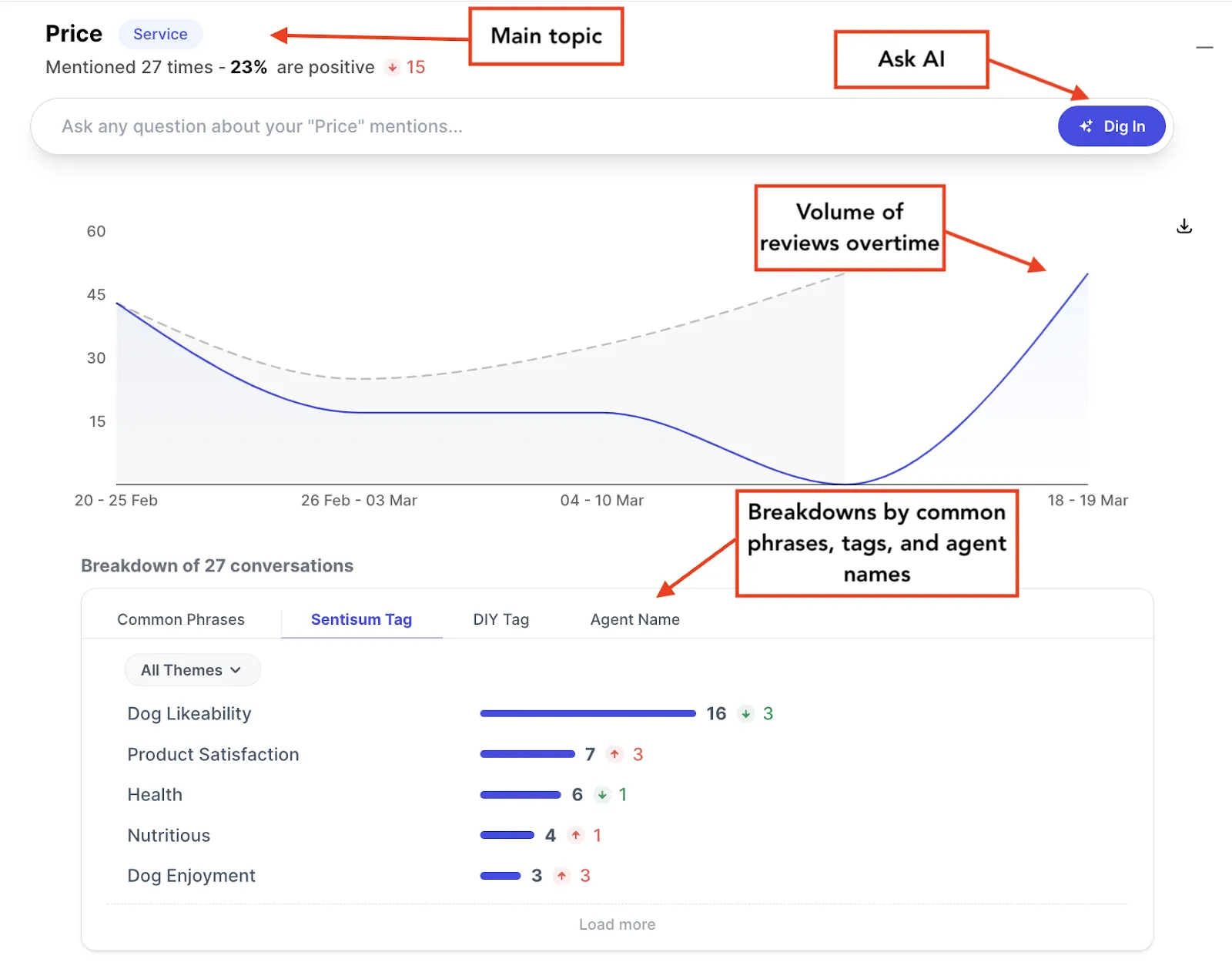
How AI sentiment analysis on reviews improves CX
✅ Improved product development: Organizations can make informed decisions about product improvements with key themes identified.
✅ Enhanced brand reputation: The ability to understand and address negative reviews promptly can enhance overall brand reputation.
✅ Increased sales: Understanding the drivers of positive and negative reviews can help your team double down on what’s working and make targeted improvements to solve customer pain points.
This, in turn, will lead to more satisfied customers who leave positive reviews.
BrightLocal data shows that customers will spend over 30% more with a business when it has excellent customer reviews.
📖 Read more: How to Conduct Sentiment Analysis Reviews [2 Methods]
5. AI Sentiment Analysis on Support Tickets
Most traditional support ticket processes leave important feedback unaddressed due to the volume and unstructured nature of the data.
AI sentiment analysis software like SentiSum integrates with help desk software like Zendesk, Freshdesk, Dixa, and others to aggregate trend data on ticket volume, frequent topics, and top increases and decreases over time.

In addition to providing a high-level overview of support ticket trends, SentiSum labels each conversation based on the core issue(s), such as technical problems, billing inquiries, or service requests.
You can also view the urgency of each support ticket to help your team tackle the most pressing issues first, based on custom triage and prioritization workflows that you can establish in SentiSum.
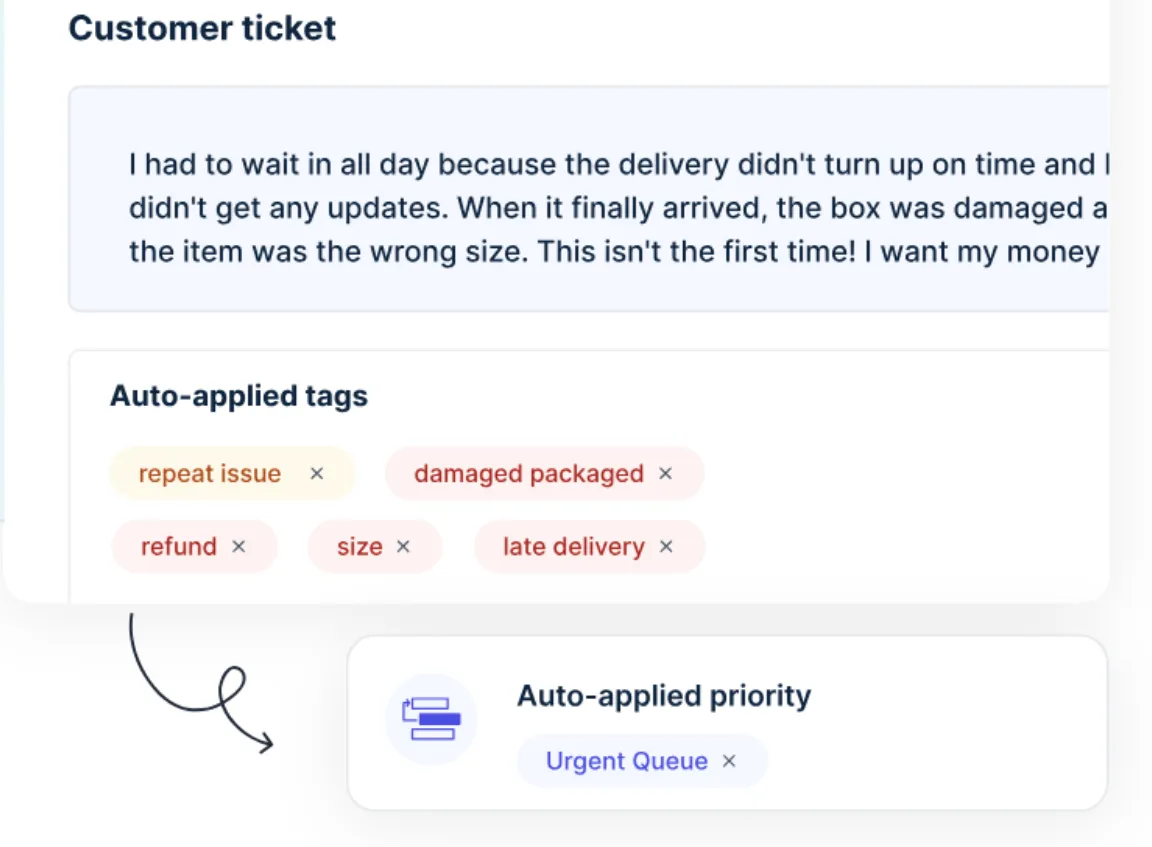
These insights are then pushed back to your help desk software so you and your agents have the same information across platforms.
How AI sentiment analysis on support tickets improves CX
✅ Reduced resolution time: James Villas reduced its first reply time on support tickets by 46% in just a few weeks using SentiSum’s AI-powered insights.
"Sentisum helps us to organise our tickets and triage them to the right team member with the necessary skills to solve a ticket with the best possible outcome,” said Johannes Ganter, Head of CRM at James Villas.
✅ Proactive issue resolution: Deep insights into which support ticket topics are on the rise can help your team address major problems faster and improve overall service quality.
✅ Targeted product improvements: Knowing which product features keep coming in support tickets can help you collaborate with your product team to improve those features or roll out new ones to meet customer needs.
📖 Read more: Zendesk Ticket Analysis: 2 Ways to Analyze Support Ticket Data
How *Real* Companies Use AI-Powered Customer Sentiment Analysis Tools to Drive Business Results
Still not sure how AI-powered customer sentiment analysis can help you create tangible, meaningful change in your organization?
Here are a few real-world examples of how companies have used sentiment analysis tools to improve the customer experience and drive results across departments.
1. Seamlessly Integrate All Customer Feedback Sources
Before implementing AI-powered sentiment analysis, Butternut Box faced challenges with fragmented customer feedback.
The company relied on traditional methods that gave a limited view of customer insights and left untapped valuable data from various feedback channels.
Butternut Box wanted to unify its customer feedback sources, including solicited and unsolicited feedback from surveys and customer support tickets, to gain a more comprehensive understanding of customer sentiment and experiences.
With SentiSum, Butternut Box seamlessly consolidated feedback from all channels onto a single platform.
This integration bridged the gap between manual survey analysis and untapped customer conversations for a more holistic view of customer sentiment.
Automating the analysis process eliminated the human biases in manual analysis and delivered more accurate and consistent insights.
AI-driven insights from SentiSum allowed Butternut Box to transform its customer feedback into actionable strategies.
This approach has led to successful brand campaigns, strategic changes in product offerings, and a heightened focus on delivering value to customers, all of which have contributed to the company’s expansion into new markets.
Learn more: Webinar: Empowering Teams Through AI-Driven CX Insights
2. Reduce Customer Support Response Times
Deliverr (now Shopify Logistics) struggled with long response times from its support department.
This not only impacted overall customer satisfaction but also significantly strained the support team’s relationships with other departments.
When Justin Rezende, Head of Support at Shopify Logistics, began exploring AI-powered solutions for Deliverr, his goal was to understand what exactly was causing such long response times so his team could optimize its support processes accordingly.
With the help of AI sentiment analysis, Deliverr was able to identify the primary culprit: a lack of resources.
Thanks to the insights uncovered by AI, the support team was able to pinpoint common issues and prioritize resources more effectively to address the most urgent customer issues first.
This simple shift helped Deliverr reduce response times by over 90%, which had a ripple effect across the organization.
The improved customer satisfaction from shorter support wait times also led to higher CSAT scores, cost savings from more efficient use of support resources, and better collaboration between the product and support teams.
🎙️ Podcast: Reducing reply times by over 90% at Deliverr
3. Align Product Roadmaps with Real Customer Feedback and Prevent Churn
With a high volume of support tickets, Scandinavian Biolabs struggled to identify the top issues that were causing customer satisfaction and churn.
This lack of insights led to operational inefficiencies and significant delays in resolving customer problems.
The company wanted to use AI-powered sentiment analysis tools to gain a comprehensive understanding of customer pain points based on its support data.
These insights would allow the entire organization to prioritize and address critical issues effectively, as well as inform its product strategies based on what customers actually wanted.
Scandinavian Biolabs integrated SentiSum with its support ticket platform and created 55 custom AI-generated tags.
This gave the team an objective view of the data and highlighted the most consistent customer pain points.
The customer support was able to take this data to the product team so they could better align product strategies with customer feedback.
Thanks to AI-powered sentiment analysis, Scandinavian Biolabs improved its overall customer experience with reduced resolution times, decreased instances of a major driver of dissatisfaction and churn, and more helpful customer self-service documentation.
These insights also influenced the reprioritization of the company’s product roadmap, leading to the early implementation of a new customer platform.
CEO Anders Reckendorff highlighted the transformative impact of sentiment analysis:
"Support tickets are incredibly important for qualitative data, providing what you can almost view as pre-churn information,” he said.
“SentiSum allows us to view this data in an easy-to-understand format with a high-level overview of granular information. All of this leads to better initiatives and a better experience for our customers."
Book a demo below!

💡Case Study: How Scandinavian Biolabs use SentiSum to reprioritise product roadmaps and identify churn drivers