Most customer service leaders we talk to feel a creeping sense that they're getting vital insights too late—or missing them entirely.
And there's a big problem with that.
Call centers hold a goldmine of data that's useful for:
- Reducing operational overhead
- Tackling customer churn
- Improving products
In short, the data hidden in call centers could help businesses grow faster and more sustainably.
Thankfully, there's an easy way to get these benefits.
→ Text analytics
Call center text analytics tools automatically extract the topics, subtopics, sentiments, and trends from interactions between agent and customer.
At Sentisum, we've implemented text analytics into 100s of call centers across the world, so we know a thing or two about it.
So, in this article, we'll share our insider secrets with you.
Expect to learn:
- What is Call Center Text Analytics?
- How Call Center Text Analytics is Used in 2024 (5 Key Use Cases)
- 4 Key Factors of a Call Center Text Analytics Software (Look for These)
What is Call Center Text Analytics?
Call center text analytics uses natural language processing (NLP) to extract insights from text-based customer intractions at scale. This includes phone call transcripts, live chats, emails, support tickets, surveys, and more.

Different text analytics tools use different types of NLP, and this can make a huge difference in how granular and accurate your insights are.
We explain more in the next section.
How Does NLP Work for Text Analytics?
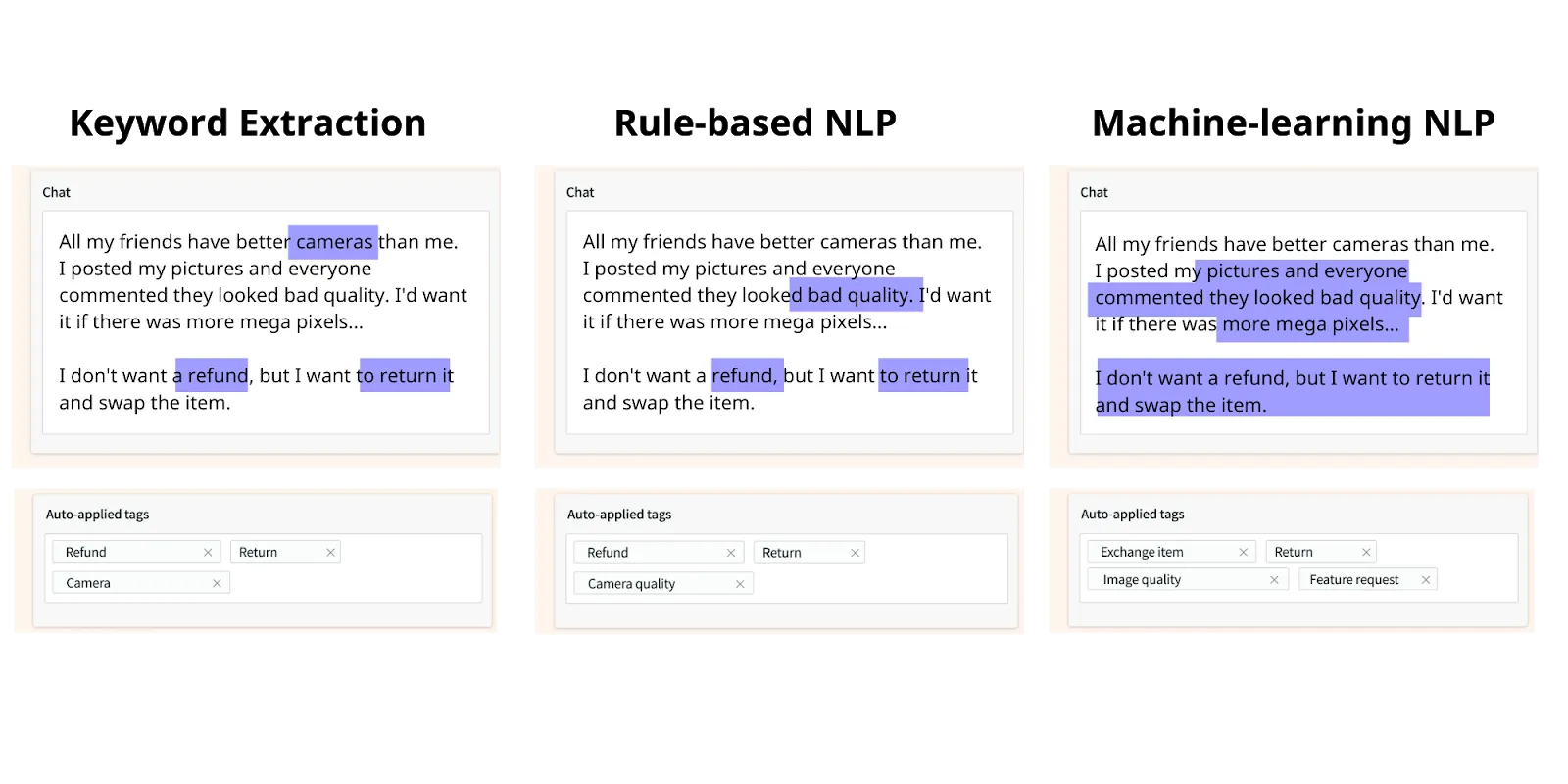
There are three main ways that NLP can be used for text analytics.
But to get granular, accurate insights, we recommend choosing a tool that uses machine learning NLP. Here’s why:
- Keyword extraction:
Most help desk tools like Zendesk or Intercom offer automated tagging based on keywords.
For example:
“If the word ‘refund’ is found anywhere in the conversation → Apply the tag ‘refund_request’ to the conversation.”
But the biggest flaw with this method is that it doesn't take into account the nuances of human language.
For instance, there are a hundred ways a customer can ask for a refund without actually using the word ‘refund’. Or the customer might use the word ‘refund’ without actually needing one.
This brings us to the next method.
- Rule-based NLP:
Rule-based NLP algorithms leverage libraries of ‘rules’ to help them understand human language.
Instead of you directly telling the automation what to do, rule-based NLP understands things like ‘liberty’ and ‘freedom’ are synonyms.
This is much more accurate than keyword extraction. But it also comes with two main downsides:
- If the rule doesn’t exist then it won’t understand the meaning.
- It’s still looking for words or sentences, rather than understanding the language like a human would.
- Machine-learning based NLP:
Machine-learning based NLP understands speech and text in a similar way to humans.
After digesting a dataset being trained in it, it uses statistical inference to carry that knowledge into new environments its never seen before.
For example, it can identify and infer the meaning of misspellings, omitted words, and new words like slang by itself.
This means it doesn’t just look at keywords or find synonyms of words. It actually understands how humans communicate and give you insights into customer sentiment, reason for contact, and more.
We built our own call center analytics software, SentiSum, using machine learning NLP. It's designed to understand and tag text data in a way that's:
1. Accurate: It tags based on meaning, not keywords.
2. Granular: Tags get to the root cause of the topic.
3. Real-time: Everything happens in milliseconds.
We apply machine learning NLP to your text data in five main ways:
5 Types of Call Center Text Analytics
To unearth accurate, granular insights, SentiSum analyses your text data in five main ways:
- Sentiment Analysis: Detecting whether text expresses positive, negative, or neutral sentiment.
- Topic Modelling: Automatically discovering main themes and topics in the text.
- Intent Analysis: Categorizing text by the intent or purpose it expresses.
- Entity Extraction: Extracting mentions of people, organisations, locations, etc.
- Keyword Analysis: Identifying key terms and phrases in customer conversations.
Let’s look at an example of how a D2C company used text analytics to get accurate insights from their customers.
Text Analytics Example: How Gousto Analysed Text Data from 9 Channels on One Dashboard
Gousto, a D2C meal kit delivery service, had nine different voice of customer channels (from AWS Connect voice calls to CSAT surveys).
Gousto needed a text analytics tool that can bring accurate, granular insights from all these channels (including voice calls) into one central platform.
The company plugged all this scattered data onto SentiSum’s dashboard.
With real-time tagging and categorisation based on machine learning NLP, Gousto’s team now has an accurate, objective understanding of customer sentiment and reasons for contact.
Joe Quinlivan, Head of Customer Care at Gousto, says:
"SentiSum helps us to significantly reduce the lead time to unearthing customer insights and sharing these across the business. We now understand these at a much more granular level which allows us to quickly put actions in place to drive improvements."

How Call Centers Use Text Analytics in 2024 [5 Key Use Cases]
“Modern text analytics tools have unlocked the call center in a way we couldn’t have imagined when we started out,” says Sharad Khandelwal, CEO of SentiSum. “The insights we now unearth at scale make it easy for every organisation to access customer data and make better decisions with it.”
Here are some creative ways for call centers to use text analytics data in 2024:
1. Text Analytics Gives You a Detailed Understanding of What’s Driving Tickets and Sentiment
Call centers deal with millions of unstructured text data from various channels. When properly analysed and sorted, this data is a goldmine of insights.
For example, SentiSum analyses two main things from your text data:
- Topic analysis: assigns topic tags or categories to text based on the underlying meaning, reason for contact or theme.
- Sentiment analysis: determines whether the text is positive, neutral or negative.
Working together, these techniques form what we call a topic-based sentiment analysis. For call centers, this is your holy grail of insights.
For instance, topic modelling can uncover frequent issues customers contact about.
This helps identify problems and conduct root-cause analysis. If laptop batteries emerge as a key topic, diagnostics can trace it back to a faulty production batch.
Sentiment analysisquantifies positive or negative feedback.
Sudden drops in NPS surveys can be connected to changes in call driver topics and sentiments. This pinpoints customer pains and guides appropriate responses.
On SentiSum, you can see both these topic and sentiment trends and details right on the main dashboard.
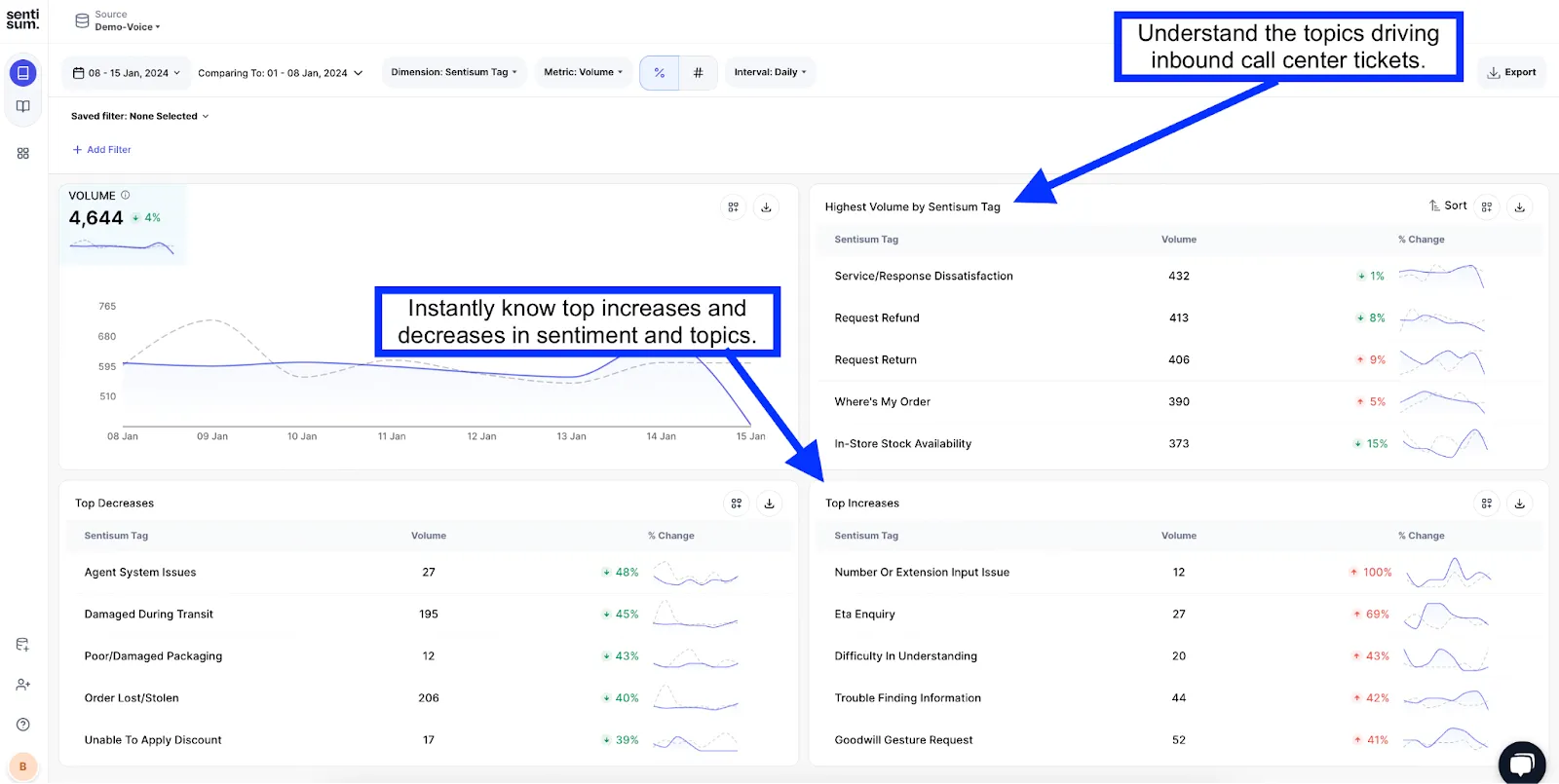
2. Text Analytics Gives You Granular Tags to Improve Triage & Prioritization
Manually tagging tickets is not only time-consuming for your agents, but also leads to inaccurate and late insights.
Instead, a text analytics tool uses machine learning NLP to auto-analyse and tag customer conversations based on sentiment, reasons for contact, and more.
These precise tags can then drive various operational use cases for call centers:
- Real-time Triage: Conversations get routed to the right agents based on difficulty, urgency, and skillset. For example, a complex technical complaint can go to a senior technician, while an information request goes to a new agent.
- Smart Prioritisation: Queues get re-ordered based on business impact. A new customer who's unable to pay can be prioritised to prevent lost sales.
- Optimisation: Historical tagging data identifies inefficiencies. For example, the UX team handling "billing errors" sees 50% negative sentiment — showing the need for a redesign.
For example, at the start of the COVID-19 pandemic, the holiday rental company James Villas reduced first reply time by 46% to customers caught in panic situations.
How? By implementing topic & sentiment analysis to identify urgent tickets and route them to a priority queue. Read more about their story here.
3. Text Analytics Gives Actionable Insight on Voice Calls
Voice calls have always been a blind spot for call centers. But with speech recognition and text analytics, you can now extract data at scale even from voice interactions.
AI can transcribe customer calls in real time with over 90% accuracy. The transcript then goes through text analytics to detect topics, trends, and sentiment.
For example, keyword analysis can reveal complaints about defective products, shipping delays, or billing errors, while sentiment analysis tells you if some customer segments have more negative experiences.
This shines a light on operational gaps and guides agent training, process changes, and service improvements. Over time, the improvements reflect in sentiment scores, closing the loop.
Our CEO, Sharad Khandelwal, says:
"Relying solely on customer reviews or surveys for insights? Think again.
Real, unfiltered feedback lives in support channels— chats, emails, calls. Analyse this data to identify truly happy customers, those on the brink, and potential brand advocates."
4. Text Analytics Gives You Easier and Faster Agent Quality Assurance
Conducting manual QA on a large sample of agent interactions can be a long and difficult task.
Previously, the call center manager would have had to sit down and listen to sample calls to analyse agent performance.
But with a text analytics tool like SentiSum, AI listens to every customer call and attaches accurate tags that describe the key issue, customer sentiment, reason for contact, and more.
This means call center managers can just read all these insights on an easy-to-use dashboard and assess agent performance. For instance, agents with unusual spikes in negative sentiment would get flagged for coaching.
Rising trends in certain complaints may suggest wider training needs. AI takes this even further by generating scorecards and recommendations based on transcripts.
5. Text Analytics Makes Fast Sense Out of CSAT Surveys and Reviews
Surveys like CSAT and NPS provide critical feedback, but analysing open-ended responses can be difficult and time-consuming.
Text analytics overcomes this challenge through automation. For example, British Airways uses Sentisum’s text analytics on NPS surveys to improve customer satisfaction.
Topic modelling highlights key problems like flight delays, food quality, or website bookings while sentiment analysis tracks if comments about these topics turn negative over time.
Correlating survey ratings to conversation topics provides deeper insight. A customer may give a low CSAT score after discussing a flight cancellation, but fail to leave feedback. But when you link these interactions, the root cause becomes clearer.
With text analytics, survey results shift from periodic reporting to continuous insight. You can spot trends before they escalate into widespread issues, guiding data-driven decisions to improve customer satisfaction.
4 Key Factors of a Software for Call Center Text Analytics (Look for These)
What features should you search for in call center text analytics software?
Kirsty Pinner, our Product Owner, talks about the things that companies look for based on her experience dealing with feature requests at SentiSum:
"What customers request is important. You should always listen. But you shouldn't (just) listen to the feature request, you should listen to the pain behind it."
Related read: 3 Best Call Center Voice Analytics Software in 2024
Factor 1: Does the Tool Analyze All Customer Feedback Channels?
Support conversations happen across different channels: phone calls, emails, chats, social media, and more.
You want a text analytics tool that analyses data from ALL these channels in one place.
Factor 2: Are the Insights Genuinely Useful and Trustable?
The best voice call sentiment analysis tool turns millions of text data into accurate, helpful insights in seconds.
SentiSum applies machine learning NLP to auto-analyse and tag each text, giving you insights into customer sentiment, reason for contact, and more.
With precise tags and categories, you can deep dive into conversations to understand nuances and verify insights.
For example, a telecom provider can filter conversations by “network issues” and “negative sentiment” to analyse complaints.
We also recently launched Dig In, which is like ChatGPT for your CX data. Ask any questions you have about a particular issue, and get succinct, summarised answers in seconds.
Factor 3: Is the Tool Easy to Use for Anyone Who Needs It?
Advanced features are helpful, but they shouldn’t come at a high training cost to you.
Complex tools create even more silos across different departments. Important insights never get implemented when stakeholders don't know how to use a tool.
The best call center text analytics software is easy to use and easy to share insights with other team members.
Anyone (not just your CX leader) should be able to just log in and start reading insights right away. This ease of use is what really drives change across departments.
“Imagine a world where every team member, regardless of technical know-how, can grasp customer insights and use them to make informed decisions.”
- Sharad Khandelwal, CEO of SentiSum
Factor 4: Does the Tool Integrate with Your Existing Tech Stack?
Your voice call data likely comes with help desk platforms like Zendesk or Freshdesk.
You want a text analytics tool that can not only bring data from your current tech stack, but also push automations and insights to them. This is crucial to set up prioritisation and triage rules for your customer support team.
On SentiSum, you can build custom automation flows with a custom AI model and push insights back to your help desk platform.

If you have more questions on call center text analytics, we answer them below ⬇️